ZONIFICACIÓN BASADA EN LA RESPUESTA DE ECOSISTEMAS A OSCILACIONES CLIMÁTICAS
Resumen
El objetivo del presente trabajo fue realizar una zonificación de la provincia de Buenos Aires, Argentina, utilizando cambios en la dinámica de su cobertura vegetal provocados por oscilaciones climáticas. Se usaron datos de 19 variables bioclimáticas actuales (décadas 1950 a 2000), otras provenientes de predicción de cambio climático según el Modelo WCCM3 (cambio global CO2x2), e imágenes satelitales Terra Modis (serie temporal 2000-2010; 23 imágenes por año) y Spot vegetation (serie temporal 2008-2010; 12 imágenes por año) con el índice de verdor (NDVI), calculado por las respectivas agencias espaciales. Las imágenes vegetation se clasificaron en aquellas provenientes de años “el niño”, neutros o “la niña”, y se co-registraron con las capas raster de variables bioclimáticas. Los datos se analizaron mediante correlaciones canónicas, definiendo como una de las matrices, a la diferencia entre los parámetros bioclimáticos actuales y los predichos por el el Modelo WCCM3, y otra de las matrices, con los valores de NDVI de tres series de datos, correspondientes a los tres estados climáticos mencionados. Posteriormente, utilizando una fuente de datos independiente como las imágenes Modis se realizaron ajustes de los datos de NDVI a las regiones delimitadas. Sobre la base de los resultados de los análisis multivariados se delinearon zonas de comportamiento homogéneo en las tres variables canónicas (92 % de correlación explicada). El análisis canónico arrojó un r de 0,92440 [chi²(450)=1334e2 p<0,0001] con autovalores 0,85 ; 0,80; y 0,64 para los tres primeros ejes canónicos. Las variables que obtuvieron mayor carga sobre los ejes de correlación fueron: máxima temperatura del mes más cálido, mínima del mes más frío y el rango anual de temperaturas. En el caso de los valores de NDVI, los meses niño aportaron mayor correlación. Los resultados obtenidos mediante el ajuste de los valores de NDVI al Modelo Timesat, muestran un comportamiento diferente de las distintas zonas en varios de sus parámetros apoyando la subdivisión realizada.
Palabras clave: Modis, imágenes de vegetación, análisis multivariado, ENOS
Abstract
The aim of this study was to conduct a zoning of the province of Buenos Aires, Argentina, using changes in the dynamics of its vegetation cover caused by climatic oscillations. Layers consisting in data of 19 bioclimatic variables (decades 1950 to 2000); bioclimatic variables in a climate change prediction by the model WCCM3 (CO2x2 global change); satellite images Terra MODIS and SPOT Vegetation greenness (NDVI) images were stored in a raster geographical information system (GIS); last consisting in a time series of 2008-2010 (12 images per year), calculated by their respective space agencies. Multivariate correlation was evaluated between climate changes predicted by global model WCCM3 and the observed climate changes by the effect of El Niño Southern Oscillation (ENSO). Geographical coherence of these correlations was visually analyzed exporting "factorial scores" product of the analysis, and then tracing the boundaries of areas on the computer screen. Subsequently, using a separate data source as MODIS NDVI; by means of the model Timesat where analyzed 7 or 8 months -depending on the case- in those years of greatest environmental contrasts, as events "El Niño "(Jun2002-March2003) neutral (July2005-Feb2006) and "La Niña" (Aug2008-Feb2009). Later was performed an ecologically homogeneous zoning using three canonical variables accounting 92 % correlation explained. The canonical analysis yielded an r of 0,92440 [chi ² (450) = 1334e2 p <0.0001] with eigenvalues 0,85, 0,80, and 0,64 for the first three canonical axes. The variables having higher loading were: maximum temperature of the warmest month, minimum coldest month and the annual range of temperatures. For NDVI values, months provided greater correlation were from Niño. The results obtained with Timesat indicate that the different zones behave differentially, supporting the main working hypothesis.
Keywords: Modis, images of vegetation, multivariate analysis, ENSOTexto completo:
PDFReferencias
Andersson L. y Sivertun A. 1991. A GIS supported method for detecting the hydrological mosaic and the role of man as a hydrological factor. Landscape Ecology 5(2) 107-124.
Beran M. A., Brilly, M., Beckery, A. y Bonacci, O. (ed.). 1990. Regionalization in Hydrology. IAHS, publicación Nº 191. 260 págs.
Cabrera, A. 1968. La vegetación de la Provincia de Buenos Aires. En: Flora de la Provincia de Buenos Aires. Colección Científica INTA 4(1): 101-134
Coops, N. C., Wulder, M. A. y Iwanicka, D. 2009. An environmental domain classification of Canada using earth observation data for biodiversity assessment. Ecological Informatics 4: 8-22.
Eastman, J. R. 2009. IDRISI 16: The Taiga Edition (Worcester, MA: Clark University).
Eklundh, l. y Jönsson, P., 2009, Timesat 3.0 Software Manual, Lund University, Sweden.
Fovell, R. G. y Fovell, M. Y. C. 1993 Climate zones of the conterminous United States defined using cluster analysis. Journal of Climate 6: 2103–2135
Frenguelli, J. 1941. Rasgos principales de Fitogeografia Argentina. Revista Museo La Plata. Argentina. Nueva serie. Botánica 3: 65-181
Gandini, M. L. y Castellar, C. 2011. Last La Niña Affects Agroecological Systems of Argentine Pampas. 34th International Symposium on Remote Sensing of Environment The GEOSS Era: Towards Operational Environmental Monitoring.
Gallopín, G. C. 1982. Una metodología multivariable para la regionalización ambiental-I. Bases Metodológicas. Ecología Argentina 7: 161-76.
Govindasamy, B., Duffy, P. B. y Coquard, J. 2003. High-resolution simulations of global climate, part 2: effects of increased greenhouse cases. Climate Dynamics 21: 391-404.
Harff, J. y Davis, J. C. 1990. Regionalization in geology by multivariate classification. Mathematical Geology 22: 573–588.
Hargrove, W. W., Hoffman, F. M. y Law, B. E. 2003. New analysis reveals representativeness of the AmeriFlux network. Eos Trans. AGU, 84(48): 529-535.
Hijmans, R. J., Cameron, S. E., Parra, J. L., Jones, P.G. y Jarvis, A. 2005. Very high resolution interpolated climate surfaces for global land areas. International Journal of Climatology 25: 1965-1978.
Host, G. E., Polzer, P. L., Mladenoff, K. J., White, M.A. y Crow, T.R. 1996. A quantitative approach to developing regional ecosystem classifications. Ecological Applications 6 (2): 608-618.
Hughes, R. M. y Larsen, D. P. 1988. Ecoregions: an approach to surface water protection. Journal Water Pollution Control Federation 486-493.
Jönsson, P. y Eklundh, L. 2002. Seasonality extraction and noise removal by function fitting to time-series of satellite sensor data, IEEE Transactions of Geoscience and Remote Sensing 40 8: 1824-1832.
Jönsson, P. y Eklundh, L. 2004. Timesat - a program for analyzing time-series of satellite sensor data. Computers and Geosciences 30: 833-845.
Kandus, P., Minotti, P. y Borro, M. 2011. Contribuciones al conocimiento de los humedales del Delta del Río Paraná: herramientas para la evaluación de la sustentabilidad ambiental. Compilado por Patricia Kandus; Priscilla Minotti; Marta Borro. - 1a ed. - San Martín: Universidad Nacional de Gral. San Martín. UNSAM Edita, 2011
Lark, R. M. 1998. Forming spatially coherent regions by classification of multi-variate data: an example from the analysis of maps of crop yield. International Journal of Geographical Information Science 12: 83-98
Leathwick, J. R., Overton J. M. y McLeod, M. 2003. An environmental domain classification of New Zealand and its use as a tool for biodiversity management. Conservation Biology 17: 1612-1623
Long, J., Trisalyn, N. y Wulder M. 2010. Regionalization of Landscape Pattern Indices Using Multivariate Cluster Analysis. Environmental Management 46:134-142
Moscatelli, G. 1991. Los suelos de la Región pampeana 1-76. En: Barsky, O. El desarrollo agropecuario pampeano. INDEC-INTA-IICA. Grupo Editor Latinoamericano. 1-804
Nathan, R. J. y Mcmahon, T.A. 1990. Identification of Homogeneous Regions for the purposes of Regionalisation. Journal of Hydrology 121: 217-238.
Ng, R. T. y Han, J. 2002. Clarans: “a method for clustering objects for spatial data mining”. IEEE Transactions on Knowledge and Data Engineering 14: 1003-1016.
Parodi, L.R. 1964. Las regiones fitogeográficas argentinas. Pp. 1-14 in ipse (ed.), Enciclopedia argentina de agricultura y jardinería, vol. 2. Buenos Aires: ACME.
Schultz, J. 2005. The ecozones of the world. Springer-Verlag, Berlin. 252 págs.
Solbrig, O. y Morello, J. 1997. Reflexiones generales sobre el deterioro de la capacidad productiva de la pampa Húmeda Argentina. 1- 28. En: Morello, J. y Solbrig, O. Eds. ¿Argentina granero del mundo: hasta cuándo? Orientación Gráfica Editora. 171-183.
Soriano, A., León, R. J. C., Sala, O. E., Lavado, R. S. y Deregibus, V.A. 1991. Rio de la Plata Grasslands. En: Copeland, RT (Ed.), Ecosystems of the World. Natural Grasslands, Introduction and Western Hemisphere. Elsevier, New York, 367-407.
Viglizzo, E., Frank, F. Y Carreño, L. 2005. Situación Ambiental En Las Ecorregiones Pampa Y Campos Y Malezales. En: Situación Ambiental Argentina. 261-278 En: Brown, A., Martínez Ortiz, U., Acerbi, M., Corcuera, J., (Eds.), Situación ambiental Argentina 2005, tercera edicion Fundación Vida Silvestre Argentina, Buenos Aires, pp. 261–269
Villar, J. y Cencig, G. 2010. Secuencias agrícolas: recurso para mejorar el uso del agua y la productividad del suelo. INTA-Estación Experimental Agropecuaria Rafaela. Información Técnica Cultivos de Verano. Campaña 2010. Publicación Miscelánea Nº 118
Enlaces de Referencia
- Por el momento, no existen enlaces de referencia
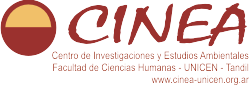